Credit Scoring in Kenya: observations and comparisons from the field
August 30, 2012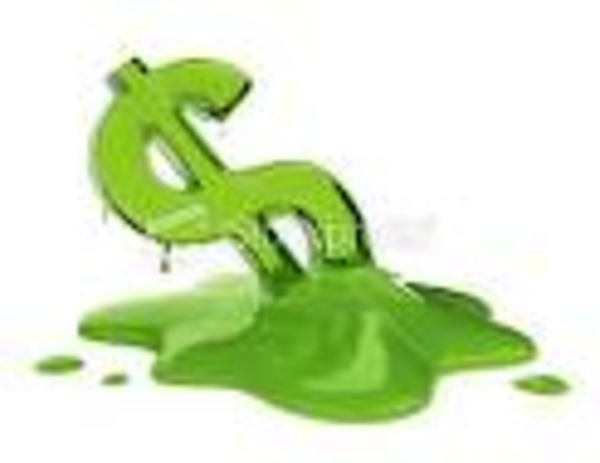
My Fellowship workplan has been focused a little more on the technical side of things, with more application programming and appraisal analysis than borrower verifications. From such projects, and also because I come from a banking, lending and risk management background, it seems fitting to at least put forth some observations regarding the use of credit scores across the Kenya micro landscape. However, the way we approach credit scoring in the USA is almost opposite from current practices here, where aggregated financial data at the individual level could still be years away.
Such a difference does not imply that Kenyan credit evaluations are less accurate, especially after reflecting on USA’s recent financial meltdown, where most credit bureau and banking models completely failed. Each country’s process thus has pros and cons, but either way it’s almost certain that institutional credit scores in Kenya will emerge quite differently from what we have now in USA. I met a sales rep here who had just come from a job at a major credit bureau and his assessment was the same – the application of ‘Western’ models to sub-Saharan borrowers simply didn’t work.
So why not, and what are the specific contrasts in Kenya?
First, most applications here are done on paper at the farm, not with real-time data entry at the branch. Loan officers then manually evaluate each application based on thorough knowledge of the product, region and industry, which tends to yield a good decision, but this method is obviously less scalable and depends on lengthy training. In the USA, personal bankers are a dime a dozen, with most training focused on how to use the systems, usually enabling a high volume of originations but only mediocre knowledge of the product suite.
Second, there are very few sophisticated data models here. In the US, we have gotten used to ‘instant’ credit decisions that are based on systems requesting a bureau score in real-time, followed by proprietary models calculating an application score: approve / decline or red / yellow / green. All of these systems and models require large amounts of data, and in Kenya, organized data is still pretty sparse. Sometimes even key application metrics never make it from paper into the MIS repository, limiting all down-stream modeling capability.
Third and most importantly, one of microcredit’s strongest risk management practices is a requirement that each borrower be part of a group. When used, this creates a community that both spreads the risk and invokes peer pressure to help with repayments. Some proponents claim that groups are the very cornerstone of successful microfinance, and from what I’ve seen in Kenya, this policy directly mitigates default risk.
An interesting question is whether a group lending requirement would work in the USA, to which I would say ‘hopefully’, if we can only get past the stigma around joint liability. This is definitely something US culture could learn from Kenya – how to work together more closely and use community as a means to overcome individual barriers. Nonetheless, since Kiva is already working in New Orleans, some of you readers might be interested in this article: “Parallels Between Group Lending Communities in the Developing World and a Post-Katrina New Orleans”
And here’s something Kenya might need to consider at some point: one of the reasons US policies favor models is because systematic decisions can exclude inputs that might cause discrimination. In Kenya, some credit officers favor female applicants, and pioneer Grameen Bank lends 97% of its funds to women. The reasons behind this are legit – studies exist that support women as more likely to repay and more likely to apply surplus income to the household or school fees – but plain and simple, any lending practice in the USA that discriminates based on gender is prohibited.
To overcome the ‘data barrier’ I built a rudimentary data set with about 250 appraisals from the field. From there I was at least able to use R and run a decision tree model to generate a few possible indicators. Even major US banks run d-trees, because they are easy to explain to regulators and easy to implement, and I think Kenya microcredit is close to widespread use of this model. In the US I was building neural networks, which are extremely powerful but require vast amounts of data. Kenya’s data collection and warehousing is not quite ready to harness that kind of power, but from what I heard, many leaders in the industry know the potential and want to get there.
Given the above considerations, what does that possible future look like?
The two credit scoring companies I met with during my 3 month Fellowship both said the same thing – their model will produce an accurate credit score only after the MFI requires its borrowers to start transmitting all income and expenses on a weekly basis or all milk sales on a daily basis. This need for more data, for heavy (mobile) input way out in the field, is a recurring theme. Adoption won’t be quick – ongoing financial text messages are a lot to ask from borrowers who are just starting to understand credit! Yet these companies have the right idea and are already forming the vague future of credit scoring in Kenya microfinance, where things are moving, albeit slowly, in that direction.
Varick Schwartz is a Kiva Fellow serving in Nairobi, Kenya and working with Juhudi Kilimo. You can view Juhudi’s loans on Kiva here!
PREVIOUS ARTICLE
Kiva Innovations: Tackling energy poverty in sub-Saharan Africa →NEXT ARTICLE
Passport Series: Beating the odds in Sierra Leone one loan at a time →